Week 13 Overheads
Modelling Navigation in Computer or Robotic Agents
Rodney Brooks and Company at MIT
Hannibal
A robot patterned very closely on insects.
Pebbles
Pebbles navigating in an outdoor environment.
Pebbles avoiding a downed...obstacle.
Ants
Front and back view of an ant robot. Note that thexe robots are about 3 cm long (take this link for a better idea of the scale).
A lot of sensors, emitters, and detectors are packed onto an ant robot.
An example of behaviour in a group of ants. In the figure on the left, the centre ant has found a "food item". When this ant picks up the food in its mandibles it emits an "I've found food!" signal that the other ants in the surrounding area can detect. In the figure on the right the other ants move in to see if there is any more food available.
Yamauchi and Beer
ELDEN system
Evidence grids
Correct for dead reckoning errors
Topological Mapping
Grids and Voronoi diagrams
Regions and topological graph
Pruned regions and topological graph
Wittmann & Schwegler (1995)
Are shortcuts necessary for ant path integration?
Simple, logical assumptions
Compass orientation
- Circular array of dedicated neurons
Distance
- Firing rate of neuron "clock"
Short-term memory store
Accurage path integration
Alternate solution?
Waterstrider Prey Orientation Model: Snyder (1998)
Simple artificial neural network
---Insert Image---
Baseline
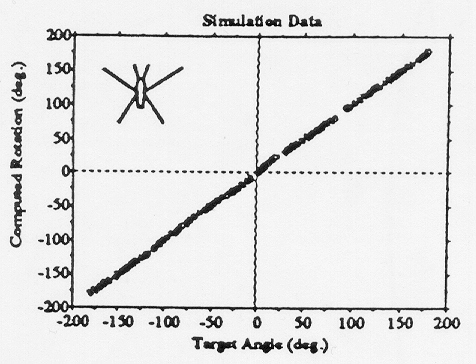
Amputations
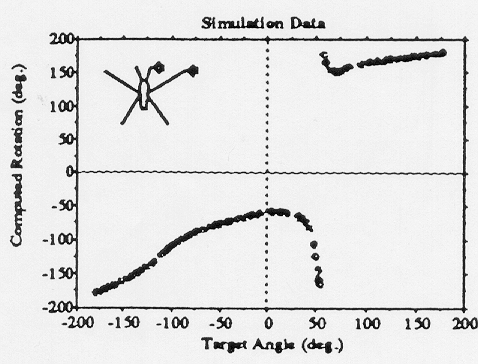
Relearning?
Performance of the ANN when the receptors of the first and second right legs are lesioned (left) and after re-training (right). Notice that prior to re-training the ANN makes the expected systematic errors in rotation (i.e., rotations to the left instead of the right within the angular range covered by the lesioned receptors). Following re-training the lesioned network performs as would be expected if it was intact.
Return to 403 course page.